OUTCOMES
Agilitas successfully launched, reducing the food reformulation process from months to weeks with AI-driven features.
3
paid multi-year customer contracts
10x
growth in active users, from 10 to 100s
75%
of users completed primary task of uploading and modifying a formula
FIRST HYPOTHESIS
We hypothesized that an ingredient comparison tool would save food scientists significant time in their workflow, modernizing the ingredient analysis process.
The ingredient comparison tool is comprised of two components:
Summary
An overview of the table, including a summary of what to watch out for when choosing ingredients to substitute in the formula.
Comparison Table
Compare attributes of up to 4 ingredients, specific details of each are extracted from uploaded ingredient specification documents.
PIVOT TO SECOND HYPOTHESIS
Based on the research insights, we pivoted to a hypothesis that an outcomes-based formula modification tool would expedite the food development process.
The first iteration post-pivot.
Jumpstart prompts
Food scientists can make AI-assisted changes using common formula modifications.
Manually modify formula
Providing the option to make manual changes to the formula, including the ingredients list or proportions
Nutrition Label
View how the changes to the formula impact the end-result nutrition label.
After a few rounds of usability testing, there were 4 key changes to the product experience.
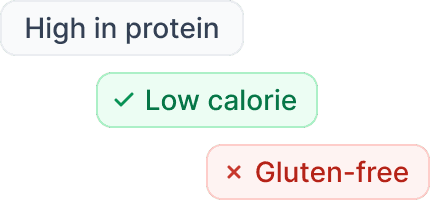
Prioritize project goals
As part of the project brief, food scientists have to achieve nutritional or regulatory targets in their formula.
The formula targets is at the very top so that food scientists know what their targets are, and whether that specific formula version meets those targets or not.
Version control
Each formula goes through multiple versions, with tweaks in the ingredients, proportions, or preparation steps.
Food scientists like to toggle between different versions to view what has been changed from version to version, so that they know whether they are closer to their formula targets.

Open-ended chat with AI
In the first version, users were completely skipping over the jumpstart prompts.
Rather than constraining to specific types of modifications, an open-ended chat aligns with current AI design principles and supports a wider range of modifications.
Support for multiple units
Although grams is the most common unit of measurement, food scientists who work with beverages work in mL.
Separating the quantity and the unit supports formulas that have more than one unit of measurement.
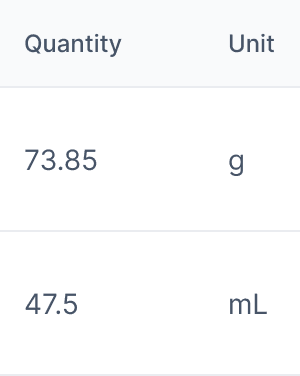
The final iteration post-pivot.